The current technique for prostate cancer detection is B-mode transrectal ultrasound (TRUS) guided systematic core needle biopsy. However, due to the limited sensitivity and capability of categorizing aggressiveness of prostate cancer in B-mode images, additional imaging modality such as magnetic resonance imaging (MRI) is necessary to support targeted biopsies. To reduce the imaging cost, we consider using a quantitative ultrasound imaging method, i.e., speed of sound reconstruction using ultrasound tomography (UST), with two ultrasound transducers ready for TRUS to take the place. The major challenge is the physical constraints posed by prostate’s anatomy, that full-angle ultrasound tomography is difficult because of the pubic bones. Therefore, we propose to reconstruct the UST images with limited angle, and employ deep learning to enhance the image quality.
The first approach is end-to-end deep learning1. In this work, a network with deep fully-connected layers is leveraged to encode the time-of-flight map into a lower dimension representation, then decode into the speed of sound image. Simulation results demonstrated feasibility of limited angle UST reconstruction using such deep learning technique.
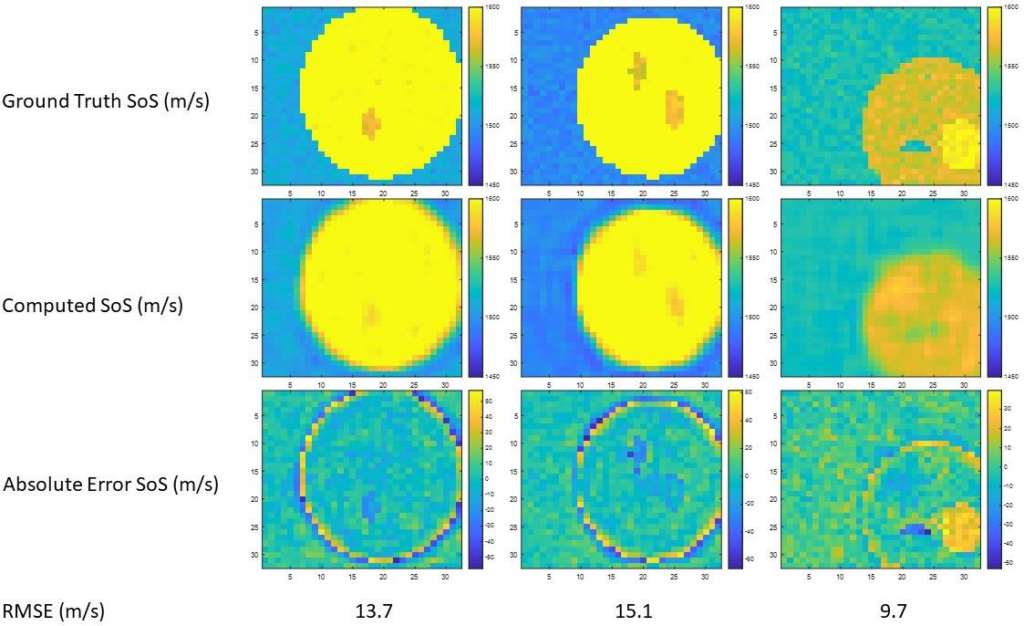
The second approach is to use the neural network as the high-pass filter in filtered back projection2. Instead of exploiting deep learning to handle the entire reconstruction task, this work specifically assign neural networks to learn the optimal filtering for back-projection. A convolutional neural network was applied for both high-pass filtering and artifact removal. By training on 5,000 and testing on 1,750 images, an average of 2.1% mean absolute error was achieved.
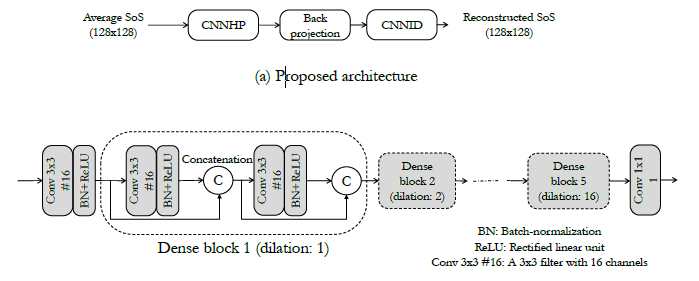
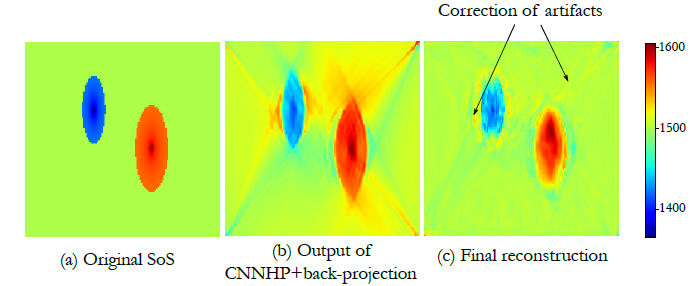
- Cheng, Alexis, et al. “Deep learning image reconstruction method for limited-angle ultrasound tomography in prostate cancer.” Medical Imaging 2019: Ultrasonic Imaging and Tomography. Vol. 10955. International Society for Optics and Photonics, 2019.
- Anas, Emran Mohammad Abu, et al. “CNN and back-projection: limited angle ultrasound tomography for speed of sound estimation.” Medical Imaging 2019: Ultrasonic Imaging and Tomography. Vol. 10955. International Society for Optics and Photonics, 2019.